Quality Data Chapter 7: Creating an Ecosystem of Quality
Data users and their needs come in all shapes and sizes, so there’s no one right way to measure or manufacture quality
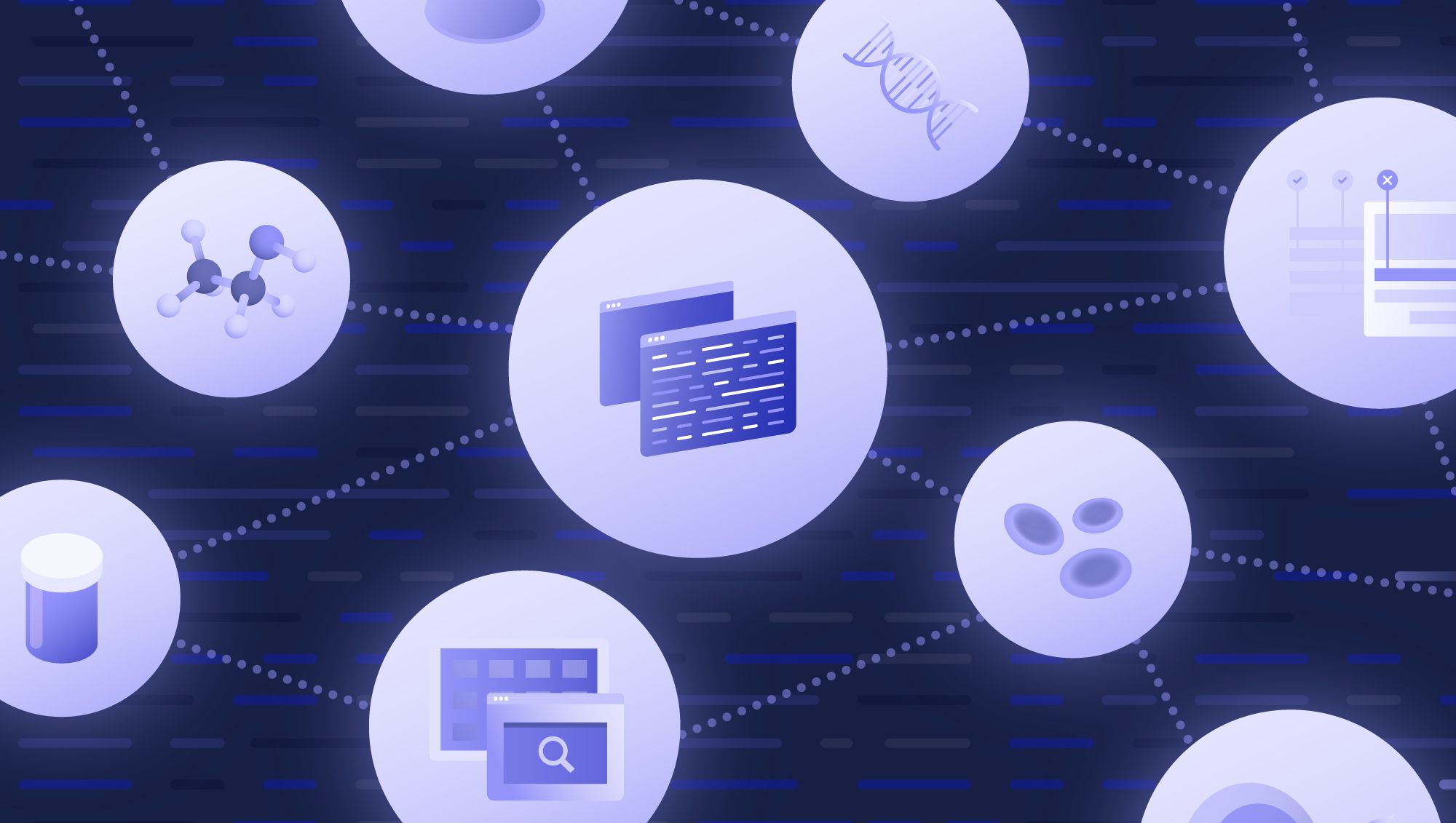
Over the course of this data quality blog series, we tapped into the deep pool of expertise here at DrugBank and have come to a very simple conclusion, defining quality in data is difficult. Fortunately, we were up for the challenge of nailing down this somewhat slippery, amorphous problem. As we pursued a concise definition, we found that our convictions that data quality must be individualized and audience-specific only grew stronger.
It is for this reason that in each blog we’ve reiterated time and again that the individual dimensions aren’t the be-all-end-all of quality. Quality isn’t even the sum of these dimensions. Yes, they should all be present, but it is having the right balance depending on any one user’s needs that results in quality.
Since we kicked things off we’ve explored the importance of:
Coverage and consistency
We discussed how quality data must have an appropriate range of information, and it must be organized in a reliable and usable fashion.
Common data entities and cross-references
Here we looked at the importance of connections between different datasets and how the presence of connections alone isn’t enough to make high-quality data. Instead, we need to look critically at how extensively the data is connected. More connectedness means a greater ability to uncover novel insights.
Hierarchies
Hierarchies in data are a necessary organizational tactic that enables more intuitive interpretations and creates more flexibility for the end user to manipulate and explore within.
Structure
How data is structured, or pre-interpreted and laid out, can make a huge difference on usability. Structured data increases flexibility and can be incorporated more quickly into existing models.
Evidence-based data with data-lineage and meta-data
Data that can’t be verified is useless. For that reason, we believe in maintaining rigorous standards to ensure data is rooted in evidence and that that evidence can be traced back to see where changes and shifts took place.
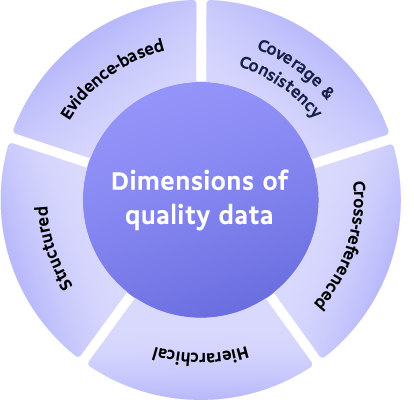
These five dimensions come together to create extremely dynamic, quality-rich data. Depending on a user’s needs, they may favour certain dimensions more than others, but ultimately the whole is worth more than each individual part. Consider that common data entities and cross-references are key for someone looking to make discoveries by combining many sources of information, whereas structured data might be more important for an ML practitioner looking to build a supervised learning model. The five dimensions create an ecosystem of quality even if the user is prioritizing specific metrics in their own work.

Our hope is that this framework makes it easier to assess the quality of your own data or the data you are considering integrating into your work. We suggest starting this examination not by looking at the data you are interested in, but by closely assessing your needs and priorities first. Then you can more confidently measure each dataset or data source against your needs.
Ask yourself:
- What dimension(s) of quality are most important to my work or specific project?
- How does this data deliver on those needs?
- How well will this data continue to deliver on those dimensions into the future?
Once you’ve found data that meets your needs, start to investigate its reliability. When it comes down to it, if you can’t rely on your data, your work will suffer. We suggest digging into sources to understand where the data comes from, who collected it, how they are dealing with biases and conflicting information, and how regularly they are updating it. Without these pieces, it is easy to slide into unreliable, conflicting, or outdated data that could discredit any findings or decisions you make.
We know that for many teams, it makes sense for them to handle their own data. However, if you’re finding that building and maintaining data is occupying more of your time than actually doing the work you set out to do, you might benefit from finding a trusted partner to lighten the load.
Partnerships can take on many different configurations. Some might need a partner that can handle all of their biomedical information, but for others, it might make more sense to approach the partnership from a hybrid perspective. If you have ample in-house expertise maybe there are some gaps in knowledge that external datasets could fill. No one source can be the gold standard, so it will often be a matter of building connections between several datasets. We have built the DrugBank Knowledgebase in a way that can easily act as a backbone for our users to easily integrate multiple sources of in-house data.

At DrugBank, we aim to take care of all the behind-the-scenes parts so that our users can focus on what they do best, without wasting their time and money fussing with data collection and organization. Every day we work alongside a wide variety of users around the world to help push the limits of biomedical knowledge. Our users range from pharmaceutical and academic researchers, clinical software developers, as well as members of the public who are able to access our data through DrugBank Online, our free-to-use website.
We’ve seen pharmaceutical teams speed up their drug discovery process from 12 years down to 3 months, clinician software developers put knowledge in the hands of prescribing physicians to manage drug-drug interactions with confidence, and we’ve even heard from DrugBank team members that their aunts and uncles have landed on go.drugbank.com and learned something new about their medications. We aim to put the power into the hands of those of you who can do the most good. But don’t take our word for it; check if we measure up by using our tools to see if we’re the right partner for you.
This blog is part of a bigger series. Missed part one? Check out the blog post here or Download Your Guide to Quality Drug Data to get the whats, whys, and hows of quality drug data, according to our experts.