Quality Data Part 5: Structured
Making it easy to find meaning amongst the chaos.

This blog is part of a bigger series. Missed part one? Check out the blog post here or download our eBook that includes parts one through four.
Quality data will never be the result of a single metric or of executing on one dimension of quality perfectly. Rather, it is the sum of many crucial elements working together. Quality is also flexible depending on different users’ needs. It is for these reasons that we take into careful consideration so many elements of quality.
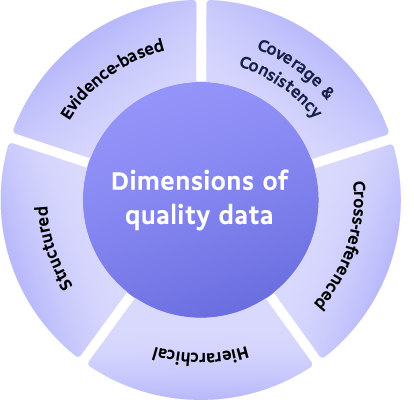
Structured Data
What is it?
To understand structured data it can be useful to start with what data looks like when it isn’t structured. Most unstructured data is simply raw text that requires interpretation for it to be used, however it can include many other mediums of information such as audio and video files. In this format, it can be efficiently exchanged between people, but computers would find it challenging to work with.
Structured data refers to information that has been organized and classified in a specific way, making it easy to access and process. It can also be thought of as pre-interpreted with key facts and points pulled out. The user is then only responsible for deciding which pieces of data to work with, and what to do with them.
Consider the process of making a cake. An analogy for structured versus unstructured data would be a recipe card versus a how-to video.

You could go online and watch a quick step-by-step video that would help you understand the overall process and what types of ingredients you would need to make a cake. But in order to actually make the cake, you would need to interpret the video to get a specific list of ingredients, then figure out measurements, timing, temperatures, and the order of operations. Although you can learn to bake a cake in this way, it would require a high level of interpretation. You also wouldn't be able to take a playlist of cake-baking videos and easily pick out a recipe that matches the ingredients and tools you have on hand. To do that, you would have to watch each video and figure out the process one by one.
In a recipe card, all that legwork would be done for you and you could simply follow along the clearly organized format, checking back whenever you needed to. This structured data removes the need for the user to do any interpretation making it an easier process to successfully bake a cake.
The structured drug data provided by DrugBank works similarly. Different types of information about drugs are classified and organized in a way that is easy to find. This allows clinical and pharmaceutical companies to build their own solutions, academics to do vital research, the general public to easily access information, and individuals in clinical care to make decisions with confidence.
How do we do it?
The structure of data is like the design of an object. The handle on a kettle enables and encourages you to pick it up. By following the design, you’re more likely to use it correctly and avoid burning your hand. When we create a data structure, we approach it in much the same way. We consider how our users want to be able to manipulate our data as well as what uses and applications we want to encourage or make easier. Then we structure our data in ways that will ensure these uses are as simple as possible.
To turn unstructured data into structured data we rely heavily on our curation and development teams, which are stacked with subject matter experts. These teams work alongside one another to identify and define common data entities and attributes for different drug datasets (attributes such as drug name, patient characteristic, route, dosage, and form). This process involves investigating and analyzing drug data to determine how it can best be connected and cross-referenced. Our team is also always reassessing and establishing strict curation standards to ensure that all our structured data is consistent.
Let’s look at a few examples of structured data that we maintain:
Indications, contraindications, and adverse effects
DrugBank's users can view the indications, contraindications, and adverse effects for every approved drug. Instead of seeing them as text descriptions (unstructured data), indications, contraindications, and adverse effects are structured in their simplest terms, without losing the level of detail that is provided in a text.
Drug metabolism
This dataset allows people to see the different steps involved in the metabolism of drugs. When a drug is degraded by the body, it produces different compounds or metabolites that may or may not have an effect on a person. DrugBank associates metabolites in an organized manner and maps them to other sets of information such as pharmacology, drug targets and other drug-protein relationships, and SNP data.
Drug-drug interactions
When a patient takes more than one drug, there is a possibility that the two will interact with each other. With drug-drug interactions, it is important to know what the severity of the interaction is, the reason why it happens, and how it needs to be managed. DrugBank has structured this information so that it can be easily queried.
Why should you care?
Structured data is important from both a user’s perspective and for what it allows us to do on the backend of our knowledgebase.
For us, structured data is foundational and key to maintaining many of our dimensions of quality data. Structured data is built around common data entities, making it easily cross-referenced and interpretable, and it also encourages consistency and lets us easily measure and improve on our coverage.
Further, structure enables us to do validations and ensure quality and usefulness at the time of data creation. If we determine that a property is very important to our customers, we can require it in all instances of that data structure. If we determine that a property can be expressed with a limited vocabulary without losing any value, we will favour this approach as it reduces the burden of interpretation.

The data’s structure must align with the function it will be used for, otherwise, it will be difficult to use, easy to misinterpret, and cause frustrations. Carefully structuring our data ensures we are able to deliver a highly usable product that reduces the number of decisions a user needs to make in order to work with the data.
For scientists and software developers, structured data is simply more usable than unstructured data. It is easy to integrate with existing data and systems, develop with, and requires less time to implement, which saves money.
Structured data is also ideal for software integrations including artificial intelligence, machine learning, and algorithm development. Because it maintains such a strict format, structured data provides a great deal of flexibility for users to manipulate it in ways that meet their unique needs. Whether that is using structured indications data for clinical decision support or as a convenient drug-drug interaction tool.
It also makes connecting to external datasets, ontologies, and resources (such as ICD-10 and UniProt) very easy. The more structured a dataset is, the more straightforward it will be to build relationships between drugs, drug products, and conditions. Ultimately this makes it that much easier to incorporate all the relevant data one might need for their research or into their decision making processes.
Final Thoughts
DrugBank understands the value and versatility of structured data. We have organized drug information in a way that is easy to find and retrieve, keeping in mind the different applications and tools our users work with. By structuring data, we improve consistency and connectivity, and therefore, its quality.
Check out all the blogs in this series:
Part 1: Our Philosophy
Part 2: Coverage and Consistency
Part 3: Cross-referenced
Part 4: Hierarchical
Be sure to check back next month when we'll be talking meta-data and data lineage.